News Story
He Lab Taps Machine Learning to Improve Cell-Based Medicine
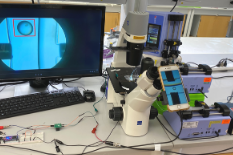
University of Maryland (UMD) Fischell Department of Bioengineering (BIOE) Professor Xiaoming (Shawn) He and members of his Multiscale Biomaterials Engineering Lab have developed an affordable system that uses machine learning and smartphone technology to improve how living cells are identified and sorted for applications in cell-based medicine. Their technique – highlighted today in Small – has the potential to enhance cell and tissue engineering and transplantation, and thus improve treatment options for a range of diseases including diabetes and infertility.
“For decades, bioengineers have applied microfluidics techniques to encapsulate cells and tissues for use in cell-based medicine. But, the process of encapsulating living cells and tissues using today’s methods poses many challenges – including the fact that, often, the process is associated with a tedious and inefficient off-chip cleaning and sorting procedure,” He said.
Much of cell-based medicine and many cellular therapies are made possible by cell donors. A donor’s cells can be used to fight disease or address a medical challenge, but there is often a limited supply. To date, bone marrow transplants – which trace back to the 1960s – remain the most common application of cell-based medicine. But, advances in research point to a future in which cell-based therapies could be used to treat conditions ranging from type 1 diabetes to infertility.
“Some cells operate like smart systems and can produce the desired medicine in response to alterations resulting from a disease,” He said. “For example, pancreatic islet beta cells can produce insulin in direct response to high levels of glucose in the blood, such as in type 1 diabetes. As such, we know that cell-based medicine has the advantage of providing a long-term, sustainable solution for treating diseases. But, due to the rarity of donor cells and tissues, it is critical that we develop new methods to engineer cells in the most valuable way possible.”
Even more, current cellular therapy techniques carry a risk that the body might wage a negative immune response to treatment, He said. This happens when the immune system attacks the cells transplanted to treat disease as it would a foreign invader – such as bacteria or a virus.
For this reason, one of the most promising techniques for delivering cell-based therapies to targets in the body involves encapsulating cells and tissues in hydrogel microcapsules. These specially designed microcapsules allow the exchange of nutrients and small molecules – such as insulin – while minimizing the risk of an immune rejection after transplantation.
But, existing methods of encapsulating cells and tissues come with a few pitfalls. For example, when bioengineers use common techniques to encapsulate particularly rare cells and tissues – such as pancreatic islets – they wind up with few useful, cell- or tissue-laden microcapsules scattered among large quantities of empty microcapsules. This happens due to two reasons: there is a limited supply of cells and tissues, and it is very challenging to encapsulate a single cell in each microcapsule.
Furthermore, cell- and tissue-laden hydrogel microcapsules are often suspended in an oil phase after formation, while the cells or tissues inside the hydrogel microcapsules require a unique aqueous solution to enable cell culturing and transplantation. If the cells or tissues themselves spend an extended period of time in oil, they may become compromised or rendered useless. As such, current extraction methods require difficult maneuvering of microencapsulated cells from oil to the aqueous phase.
Recognizing this, He and his team developed a method to selectively move microcapsules that contain cells or tissues out of the oil phase and into an aqueous solution on-chip in less than a second.
Another major challenge to cell-based medicine is that current methods to detect cells and tissues for sorting require a process known as labeling – whereby cells are “tagged” with labels, such as fluorescent molecules, to enable their detection. Labeling may lead to decreased cell viability and can limit the ability to use the cells or tissues for most health applications. This is especially detrimental when using precious cells or tissues – such as pancreatic islets for transplantation to treat type I diabetes. In these cases, labeling should not be used at all.
Knowing this, He and his research team set out to develop a label-free method.
To do this, they applied what’s known as deep learning – a type of machine learning that uses neural networks to make predictions based on input data. He and his team employed an open-source platform called TensorFlow that is capable of detecting subtle features in images with greater accuracy than the human eye. In order to use this capability of identifying living cells and tissues, the team created a system that consists of a microfluidic device for microcapsule generation, a smartphone camera for imaging an on-chip detection area, and a deep learning model for detecting cells in real time by dynamically analyzing individual video frames. The deep learning system then “decides” whether or not a microcapsule contains a cell or tissue. If the system identifies a cell or tissue, it sends that information to a microcontroller that turns on an electrical voltage across the microfluidic channel for a short period of time, enabling selective on-chip sorting of cell- or tissue-laden microcapsules apart from empty microcapsules. The voltage applied is harmless to cells and tissues – in fact, it’s similar in nature to the kind of static electricity that causes freshly dried clothing to cling together.
“Due to the limited supply of cells and tissues, we want to use cutting-edge engineering technologies to advance microencapsulation methods in such a way that allows for the highest possible quality of cells and tissues to be used in health applications,” said BIOE Ph.D. student Alisa White, first author of the Small paper. “With recent advances in machine learning, we have the exciting opportunity to utilize this technology for an improvement in the cell microencapsulation process using a simple and highly affordable setup.”
He and his team believe their technique could one day be used to advance the microencapsulation of pancreatic islets, ovarian follicles, single stem cells, and stem cell aggregates to improve safety and efficacy of treating diabetes and infertility, as well as neurodegenerative, cardiovascular, and possibly many other diseases.
This research received funding support from the National Institutes of Health. In addition to He and White, the following contributed to the Small paper: He Lab alum Yuntian Zhang (Ph.D.), BIOE Ph.D. student James Shamul, and BIOE postdoctoral fellows Jiangsheng Xu, Elyahb Allie Kwizera, and Bin Jiang.
Along with his BIOE appointment, He holds affiliate appointments with the University of Maryland’s Robert E. Fischell Institute for Biomedical Devices and the University of Maryland Marlene and Stewart Greenebaum Comprehensive Cancer Center.
Full text of the Small paper titled “Deep Learning-Enabled Label-Free On-Chip Detection and Selective Extraction of Cell Aggregate-Laden Hydrogel Microcapsules” is available online.
Published April 26, 2021